Improving Customer Experience With Predictive Analytics In Logistics
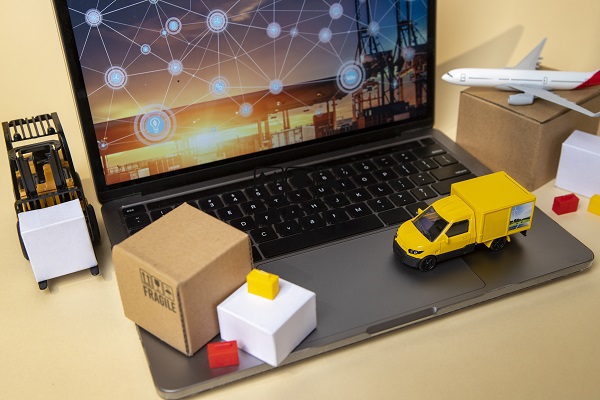
Customer satisfaction plays a pivotal role in the success of any logistics operation. In an industry driven by the movement of goods and services, meeting and exceeding customer expectations is paramount. A satisfied customer not only ensures repeat business but also acts as a brand advocate, positively influencing others. However, achieving and maintaining high levels of customer satisfaction can be challenging in the complex and dynamic world of logistics.
Through this exploration, we aim to highlight the transformative potential of predictive analytics in enhancing customer satisfaction and driving success in the logistics industry.
Understanding Customer Expectations In Logistics
Customer experience in the context of logistics refers to the degree to which customers expectations are met or exceeded throughout the entire supply chain process. It encompasses various aspects, including but not limited to on-time delivery, order accuracy, product quality, responsiveness, and effective communication. In logistics, customer satisfaction is not limited to the end customer but also extends to business partners, such as retailers, distributors, and suppliers.
Several key factors influence customer satisfaction in logistics. Firstly, on-time delivery is crucial, as customers rely on prompt shipments to meet their own deadlines or satisfy consumer demand. Order accuracy is another critical aspect, ensuring that customers receive the correct products, quantities, and specifications they requested.
Meeting customer expectations in logistics can be challenging due to several factors. The dynamic nature of the supply chain, with its numerous touchpoints and dependencies, introduces complexities that can lead to delays, errors, or miscommunication.
The increasing demands for faster delivery, shorter lead times, and personalized experiences further raise the bar for logistics companies. Balancing cost-efficiency with service quality poses a continuous challenge, as meeting tight delivery schedules while minimizing costs requires careful optimization.
Overview Of Predictive Analytics In Logistics
Predictive analytics is a powerful tool with significant relevance in the logistics industry. It leverages historical data, statistical modelling, and machine learning techniques to analyze patterns, identify trends, and make accurate predictions. By understanding and anticipating future events and outcomes, logistics companies can optimize their operations, enhance customer satisfaction, and stay ahead in a highly competitive market.
Predictive analytics in logistics starts by collecting and analyzing vast amounts of historical data, including past shipping records, customer feedback, inventory levels, and external factors like weather and market conditions. Through statistical modelling, patterns and correlations are identified, enabling the creation of predictive models. Machine learning algorithms are then applied to these models, continuously learning from new data and refining predictions over time.
This iterative process allows logistics companies to make accurate forecasts about various aspects, such as demand, delivery times, inventory levels, and transportation routes.
The benefits of predictive analytics in logistics are significant. By accurately forecasting demand, companies can optimize inventory management, ensuring the right products are available at the right locations and times, minimizing stock outs, and reducing excess inventory. This leads to improved efficiency, as logistics operations become streamlined and waste is minimized. Predictive analytics also aids in cost reduction by optimizing transportation routes, reducing fuel consumption, and optimizing resource allocation.
You may also like to read – Role Of Predictive Analytics For Risk Management In Logistics
Enhancing Customer Experience Through Predictive Analytics
Predictive Analytics For Demand Forecasting
One of the key areas where predictive analytics can significantly enhance customer satisfaction in logistics is demand forecasting. By utilizing historical data, market trends, and various external factors, predictive analytics can forecast future demand more accurately than traditional methods.
Predictive analytics utilizes sophisticated algorithms to analyze historical data and identify patterns and trends. By considering factors such as seasonality, promotional activities, economic indicators, and customer behavior, it can generate highly accurate demand forecasts. This allows logistics companies to anticipate fluctuations in demand, adjust inventory levels accordingly, and optimize their supply chain operations.
Accurate demand forecasting has a direct impact on inventory management and order fulfilment. By knowing what and how much customers are likely to order, logistics companies can ensure that the right products are stocked in the right locations and at the right time. This leads to reduced stock outs, minimized excess inventory, and improved order fulfilment rates.
Meeting customer demands promptly and efficiently significantly enhances customer satisfaction and helps to build trust and loyalty.
Predictive Analytics For Real-Time Visibility
Another critical aspect of customer satisfaction in logistics is providing real-time visibility into the status and location of shipments. Predictive analytics plays a vital role in achieving this level of visibility and transparency, benefiting both logistics companies and their customers.
Predictive analytics enables real-time visibility by integrating multiple data sources, such as GPS tracking, RFID tags, and IoT sensors, with advanced analytics algorithms. By continuously collecting and analyzing data from these sources, logistics companies can accurately track the movement of shipments, monitor their status, and predict estimated arrival times. This real-time visibility allows companies to proactively identify potential delays or issues, enabling timely intervention and effective communication with customers.
Improved visibility through predictive analytics fosters better communication and transparency with customers. By providing accurate and up-to-date information on the whereabouts of shipments, logistics companies can keep customers informed about the progress of their orders.
This transparency helps manage customer expectations, reduces anxiety related to uncertainty, and builds trust. Customers can plan their operations or adjust their schedules based on the real-time updates, resulting in increased satisfaction and confidence in the logistics provider.
Predictive Analytics For Route Optimization
Efficient and timely deliveries are crucial to customer satisfaction in logistics. Predictive analytics plays a vital role in optimizing route planning, enabling logistics companies to enhance delivery efficiency and meet customer expectations.
Predictive analytics utilizes historical data, real-time information, and advanced algorithms to optimize route planning. By analyzing factors such as traffic patterns, road conditions, weather forecasts, and delivery constraints, predictive analytics can determine the most efficient routes for each shipment. This includes identifying the optimal sequence of stops, minimizing travel distance, and considering time windows for delivery.
Through continuous analysis and machine learning, predictive analytics can adapt and refine route plans based on evolving conditions, ensuring ongoing optimization.
Optimized routes resulting from predictive analytics reduce delivery delays and contribute to improved customer satisfaction. By minimizing travel distance and considering real-time conditions, logistics companies can reduce transit times and increase the likelihood of on-time deliveries. Timely deliveries enhance customer satisfaction by meeting expectations and enabling smooth operations for recipients.
Overcoming Challenges And Implementation Considerations
Implementing predictive analytics for improving customer experience in logistics is not without its challenges and limitations. It’s important to address these hurdles to ensure successful implementation and maximize the benefits.
One common challenge is the availability and quality of data. Predictive analytics relies heavily on accurate and relevant data for accurate forecasts and insights. However, logistics data can be scattered across different systems and formats, making data integration and cleaning complex tasks. Ensuring data consistency, completeness, and reliability is crucial for the success of predictive analytics initiatives.
Another important consideration is the integration and collaboration with partners along the supply chain. Logistics operations often involve multiple stakeholders, including carriers, suppliers, and customers. Achieving end-to-end visibility and maximizing customer satisfaction requires seamless data sharing and collaboration.
Establishing data-sharing agreements, leveraging technology platforms for integration, and fostering strong partnerships are vital to ensure the smooth flow of information and optimize the benefits of predictive analytics.
To successfully implement predictive analytics solutions in logistics operations, there are practical tips and strategies to consider. Firstly, it’s crucial to define clear objectives and scope for the predictive analytics initiative. Identifying the specific areas where predictive analytics can have the most impact on customer satisfaction allows for focused efforts and measurable outcomes.
Investing in the right technology infrastructure and analytical tools is another key consideration. This includes selecting robust predictive analytics software, ensuring scalability, and providing adequate computational resources.
Future Trends
Predictive analytics for logistics is an evolving field, and there are several emerging trends and advancements that have the potential to further enhance customer satisfaction.
One notable trend is the increasing adoption of artificial intelligence (AI) in predictive analytics. AI-powered algorithms can handle large and complex datasets more efficiently, enabling more accurate predictions and faster processing times.
The Internet of Things (IoT) is another technology with immense potential to impact customer satisfaction in logistics through predictive analytics. With the proliferation of connected devices and sensors, logistics companies can gather real-time data on various aspects such as temperature, humidity, location, and condition of goods. Integrating IoT data with predictive analytics enables dynamic and responsive decision-making, allowing logistics companies to proactively address issues, ensure product quality, and deliver exceptional customer experiences.
Conclusion
Predictive analytics holds immense promise for improving customer satisfaction in logistics. By leveraging predictive analytics for demand forecasting, real-time visibility, and route optimization, logistics companies can optimize their operations, reduce delays, and enhance overall service quality.
The integration of emerging technologies like AI and IoT further expands the potential of predictive analytics for logistics. By embracing these advancements and overcoming implementation challenges, logistics companies can create a competitive edge, drive customer loyalty, and achieve sustainable growth. The significance of predictive analytics in improving customer satisfaction cannot be overstated, as it enables logistics operations to be more agile, responsive, and customer-centric in an ever-changing business landscape.